Informational Technology Courses
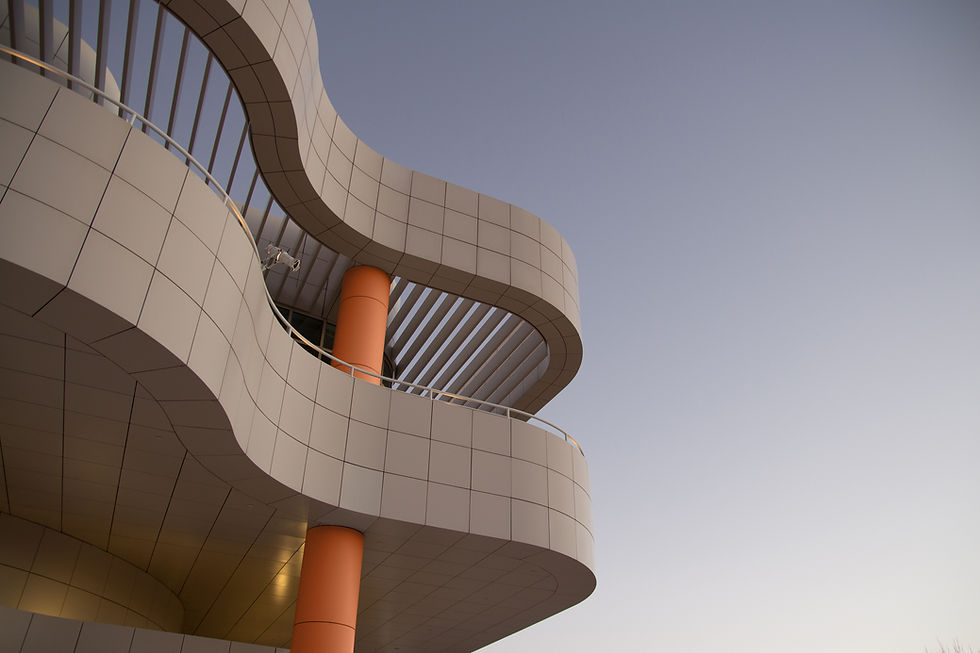
Next-Generation Data Analytics: Emerging Tools and Methods
Jun 20, 2024
5 min read
0
3

The evolution of data analytics has been one of the most significant technological advances in recent decades. With the proliferation of big data, the rise of artificial intelligence (AI), and the increasing demand for real-time insights, next-generation data analytics is transforming how organizations operate, compete, and innovate. This article explores the emerging tools and methods shaping the future of data analytics, offering insights into how businesses can leverage these advancements to gain a competitive edge.
The Evolution of Data Analytics
Data analytics has come a long way from traditional business intelligence (BI) tools and manual data processing. In the early days, organizations relied on structured data from transactional databases, utilizing basic statistical methods and BI tools for historical reporting. However, the explosion of data from various sources, including social media, IoT devices, and mobile applications, has necessitated a more sophisticated approach.
The need for real-time data processing, predictive analytics, and actionable insights has driven the development of next-generation data analytics tools and methods. These advancements enable organizations to analyze vast amounts of structured and unstructured data, uncover hidden patterns, and make data-driven decisions with unprecedented speed and accuracy.
Emerging Tools in Data Analytics
1. Artificial Intelligence and Machine Learning
AI and machine learning (ML) are at the forefront of next-generation data analytics. Computers may now learn from data and make choices or predictions without the need for explicit programming because to these technologies. ML algorithms can identify complex patterns and relationships within data, making it possible to automate tasks such as anomaly detection, customer segmentation, and demand forecasting.
Popular tools and frameworks like TensorFlow, PyTorch, and Scikit-learn are widely used for developing and deploying ML models. These tools provide powerful capabilities for data preprocessing, model training, and evaluation, making it easier for organizations to integrate AI into their analytics workflows.
2. Real-Time Data Processing
The demand for real-time insights has led to the development of advanced data processing frameworks such as Apache Kafka, Apache Flink, and Apache Storm. These tools enable organizations to ingest, process, and analyze data streams in real-time, allowing for immediate action based on current information.
Real-time data processing is particularly valuable in scenarios such as fraud detection, network monitoring, and personalized marketing, where timely insights can significantly impact outcomes. By leveraging these tools, businesses can respond to changing conditions with agility and precision.
3. Cloud-Based Analytics Platforms
The adoption of cloud computing has revolutionized data analytics by providing scalable, flexible, and cost-effective solutions. Cloud-based analytics platforms like Google BigQuery, Amazon Redshift, and Microsoft Azure Synapse Analytics offer powerful data warehousing and analytics capabilities without the need for extensive on-premises infrastructure.
These platforms support the seamless integration of various data sources, real-time data processing, and advanced analytics, making it easier for organizations to derive insights from their data. Additionally, the pay-as-you-go pricing model allows businesses to scale their analytics operations based on demand, optimizing costs and resources.
4. Data Visualization and Business Intelligence
Data visualization and BI tools have evolved to provide more interactive and intuitive ways of exploring data. Tools like Tableau, Power BI, and Looker enable users to create dynamic dashboards, reports, and visualizations that facilitate deeper insights and better decision-making.
These tools support advanced features such as natural language queries, AI-driven insights, and collaborative analytics, empowering users at all levels of the organization to engage with data. By making data more accessible and understandable, these tools help foster a data-driven culture within businesses.
Emerging Methods in Data Analytics
1. Augmented Analytics
Augmented analytics leverages AI and ML to enhance data preparation, analysis, and interpretation. This approach automates many aspects of the analytics process, from data cleansing and integration to generating insights and recommendations.
By reducing the manual effort required for data analysis, augmented analytics allows data scientists and analysts to focus on more strategic tasks. It also democratizes analytics by enabling non-technical users to gain insights from data without needing deep expertise in data science.
2. Data Fabric and Data Mesh
As organizations deal with increasingly complex data environments, new architectural approaches like data fabric and data mesh are gaining traction. A data fabric is an architecture that provides a unified and intelligent data management framework, facilitating seamless data access and integration across various sources and environments.
Data mesh, on the other hand, promotes a decentralized approach to data management, where domain teams are responsible for their own data products. This approach fosters greater ownership and accountability while enabling more scalable and flexible data architectures.
3. Explainable AI
As AI becomes more integral to data analytics, the need for transparency and explainability has become paramount. The term "explainable AI" (XAI) describes strategies and tactics that enable people to comprehend the results of AI models.
XAI tools and frameworks, such as LIME and SHAP, help demystify complex ML models by providing insights into how they make predictions. This transparency is crucial for building trust in AI systems, ensuring compliance with regulatory requirements, and facilitating the ethical use of AI in decision-making.
4. Federated Learning
Federated learning is an emerging technique that allows ML models to be trained across multiple decentralized devices or servers without sharing raw data. This approach enhances data privacy and security by keeping sensitive information on local devices while still enabling collaborative model training.
Federated learning is particularly relevant in industries like healthcare and finance, where data privacy concerns are paramount. By enabling distributed learning, this method supports the development of robust AI models while adhering to strict privacy regulations.
The Future of Data Analytics
The future of data analytics lies in the convergence of these emerging tools and methods. As AI and ML continue to advance, they will drive further automation and intelligence in data analytics, making it possible to uncover deeper insights and more accurate predictions.
Real-time data processing will become increasingly critical, enabling organizations to act on insights as they emerge. Cloud-based platforms will continue to democratize access to advanced analytics capabilities, fostering innovation and agility.
Furthermore, the emphasis on data governance, privacy, and ethics will shape the development and deployment of analytics solutions. As organizations navigate these challenges, they will need to adopt best practices and frameworks that ensure the responsible and ethical use of data.
Conclusion
Next-generation data analytics is poised to transform how businesses operate and compete. By embracing the emerging tools and methods outlined in this article, organizations can harness the full potential of their data, driving innovation, efficiency, and growth in an increasingly data-driven world. With resources available from various Data Analytics Training Institute in Vadodara, Thane, Mumbai, Noida, Delhi and other cities in India, companies can stay ahead of the curve by equipping their teams with the latest skills and knowledge in the field.