How Does Data Analytics Help in Predictive Analysis?
- Vaishali pal
- Mar 14, 2024
- 3 min read
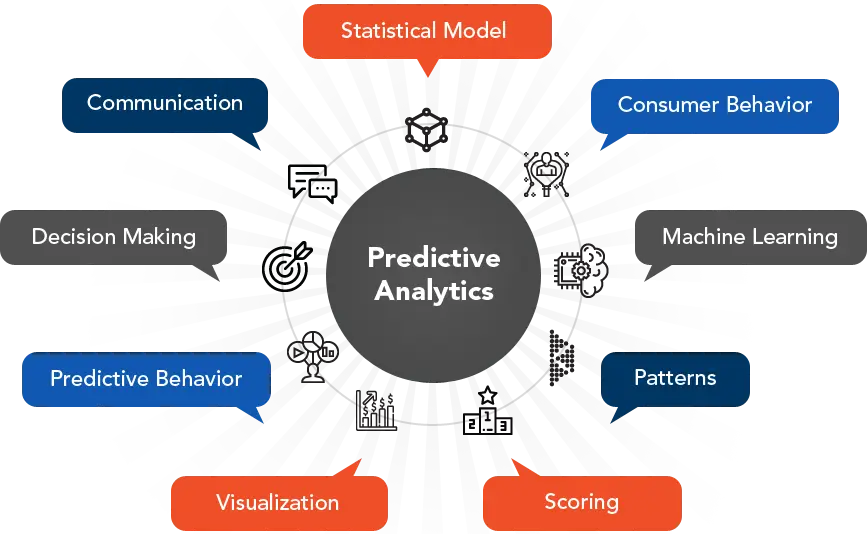
Introduction:
In the rapidly evolving landscape of technology and business, data has emerged as the new currency. The sheer volume of information generated daily has paved the way for innovative applications, with data analytics taking center stage. Among the myriad uses of data analytics, one particularly powerful application is predictive analysis. In this blog post, we'll delve into how data analytics serves as the backbone of predictive analysis and unlocks the potential to foresee future trends and outcomes.
Understanding Predictive Analysis:
Predictive analysis involves using historical data, statistical algorithms, and machine learning techniques to identify the likelihood of future outcomes based on current and past data. This forward-looking approach enables businesses to make informed decisions, mitigate risks, and capitalize on emerging opportunities. At the core of predictive analysis lies the ability to extract meaningful insights from vast datasets, and this is where data analytics plays a pivotal role.
The Role of Data Analytics:
Data Preparation and Cleaning:
Data analytics begins with the collection and preparation of data. Predictive analysis heavily relies on the quality and relevance of the data fed into the algorithms. Data analytics tools assist in cleaning and organizing datasets, ensuring that the information used for predictive modeling is accurate and reliable.
Pattern Recognition:
Data analytics employs advanced algorithms to identify patterns and trends within the data. This is crucial for predictive analysis as historical patterns often serve as indicators of future behavior. Through various statistical techniques and machine learning algorithms, data analytics uncovers hidden relationships and correlations, providing a solid foundation for making predictions.
Feature Selection and Engineering:
In predictive analysis, not all variables are equally important. Data analytics helps in selecting the most relevant features or variables for modeling. Moreover, it allows for feature engineering, where new features are created to enhance the predictive power of the model. This iterative process ensures that the predictive model is fine-tuned to capture the intricacies of the data.
Model Training and Validation:
Data analytics plays a crucial role in training predictive models using historical data. Machine learning algorithms, such as regression, decision trees, or neural networks, learn from the patterns identified during the data analysis phase. Once trained, these models undergo validation to ensure their accuracy and reliability in predicting outcomes. This iterative process, guided by data analytics, refines the models for better performance.
Continuous Learning and Adaptation:
Predictive analysis is not a one-time task but an ongoing process. As new data becomes available, the predictive models need to adapt and learn from the evolving patterns. Data analytics facilitates continuous learning by updating models with fresh data, ensuring that predictions remain accurate and relevant in dynamic environments.
Case Study: E-commerce Personalization
Consider an e-commerce platform leveraging predictive analysis to enhance user experience through personalized recommendations. Data analytics tools process vast amounts of user data, including purchase history, browsing behavior, and demographics. Through pattern recognition, the system identifies product preferences, frequently visited categories, and potential purchasing patterns.
Data analytics assists in feature selection, determining which customer behaviors are most indicative of future purchases. With the help of machine learning algorithms, the predictive model is trained and validated to make personalized product recommendations for each user.
As users interact with the platform, the predictive model continuously learns and adapts to their changing preferences. This dynamic process, powered by data analytics, ensures that the recommendations remain relevant and enticing, ultimately boosting user engagement and driving sales.
Conclusion:
In the realm of predictive analysis, data analytics emerges as the unsung hero, playing a pivotal role in enabling organizations to extract actionable insights from their data and ultimately gain a competitive edge. The process, encompassing data preparation, pattern recognition, model training, and continuous adaptation, positions data analytics as the linchpin that transforms raw data into valuable predictions.
For professionals seeking to enhance their skills in this transformative field, a noteworthy opportunity lies in pursuing a Data Analytics Training Course in Mumbai, Thane, Navi Mumbai,Vadodara, Agra, Delhi, Noida & all cities in India. Such courses provide individuals with the knowledge and expertise needed to navigate the intricacies of data analytics, ensuring they are well-equipped to contribute to the evolving landscape of predictive analysis. As the demand for skilled professionals in this domain continues to rise, undertaking a training course in Mumbai can serve as a strategic investment in one's career, opening up avenues for growth and success in the dynamic world of data analytics.