Informational Technology Courses
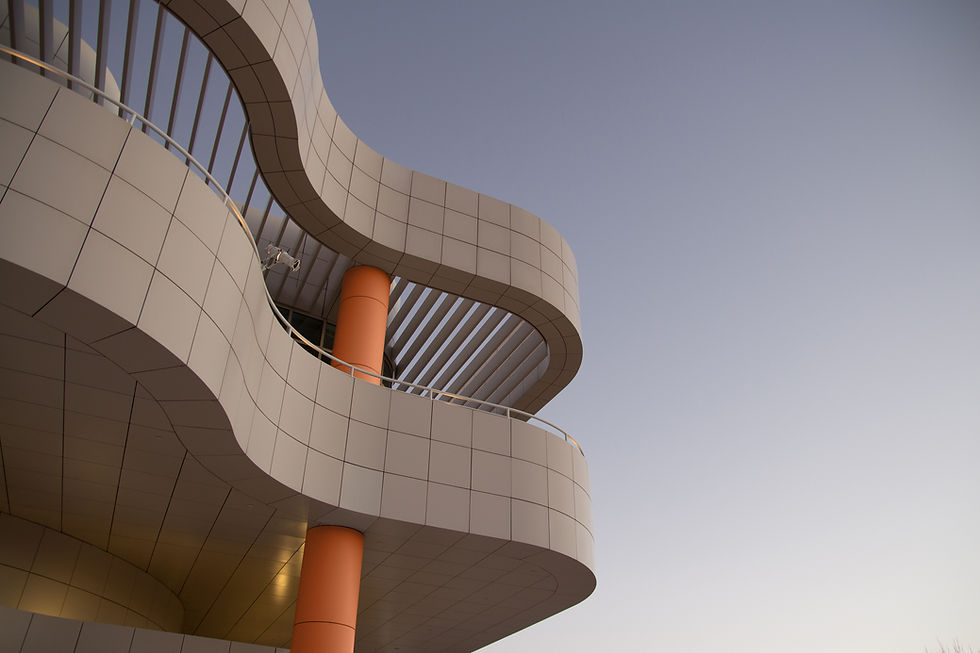
Data Analytics Tools and Software: A Comprehensive Overview
Aug 26, 2024
5 min read
0
7
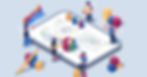
Introduction to Data Analytics Tools and Software
Data analytics tools and software are essential for transforming raw data into actionable insights. These tools enable organizations to analyze vast amounts of data, identify patterns, and make informed decisions based on empirical evidence. As the volume and complexity of data continue to grow, the role of data analytics tools becomes increasingly crucial in extracting meaningful information and driving business success.
Data analytics tools serve various purposes, including data collection, processing, visualization, and reporting. They help in managing large datasets, performing complex calculations, and generating visual representations that make data easier to understand. Choosing the right tools is vital as they impact the efficiency, accuracy, and comprehensiveness of data analysis. Effective tools can lead to better decision-making, enhanced operational efficiency, and a competitive advantage in the market.
Categories of Data Analytics Tools
Business Intelligence (BI) tools are designed to help organizations make informed decisions by providing comprehensive data analysis, reporting, and visualization capabilities. They enable users to create dashboards, generate reports, and analyze data trends in an interactive and user-friendly manner.
Key Features and Capabilities:
Interactive Dashboards: BI tools offer customizable dashboards that display key performance indicators (KPIs) and metrics in real-time.
Data Reporting: Users can create detailed reports to summarize and analyze data insights.
Advanced Analytics: Many BI tools provide features for predictive analytics and data forecasting.
Data Visualization Tools
Data visualization tools specialize in creating graphical representations of data, making it easier to interpret complex information and identify trends.
Importance:
Enhanced Understanding: Visualization tools help users grasp complex data through charts, graphs, and interactive visuals.
Improved Communication: They facilitate clear communication of insights to stakeholders and decision-makers.
These tools play a critical role in making data comprehensible and actionable, helping organizations derive insights from their data more effectively.
Statistical Analysis Tools
Statistical analysis tools are designed for performing complex statistical operations, data modeling, and hypothesis testing. These tools are crucial for researchers, data scientists, and analysts who need to perform in-depth analyses to uncover trends and relationships within data.
Key Features:
Data Modeling: Ability to create and validate statistical models.
Hypothesis Testing: Tools for conducting statistical tests to validate assumptions and theories.
Advanced Analytics: Features for performing regression analysis, factor analysis, and other advanced statistical techniques.
Applications:
Data Modeling and Analysis: Statistical tools help in building and testing models to understand data patterns and relationships.
Reporting and Visualization: They offer features for creating detailed reports and visualizations to present statistical findings.
In summary, statistical analysis tools are vital for conducting rigorous data analysis and generating actionable insights, supporting data-driven decision-making across various industries.
Big Data Tools
Big data tools are designed to manage, process, and analyze massive volumes of data that traditional databases cannot handle efficiently. These tools are essential for organizations dealing with high-velocity, high-volume, and diverse datasets, often coming from various sources like social media, IoT devices, and transactional systems.
Key Features:
Scalability: Big data tools are built to scale horizontally, allowing them to handle increasing amounts of data by adding more nodes to the network.
Distributed Processing: These tools use distributed computing frameworks to process data across multiple machines, improving performance and speed.
Examples:
Apache Hadoop: An open-source framework that uses a distributed storage and processing model. Hadoop’s Hadoop Distributed File System (HDFS) stores data across clusters, while its MapReduce component performs data processing.
Apache Spark: Known for its in-memory processing capabilities, Spark provides faster data processing compared to Hadoop. It supports various data analytics tasks, including machine learning and stream processing.
Google BigQuery: A fully-managed, serverless data warehouse designed for large-scale data analysis. It offers high-speed querying and real-time data analysis capabilities.
Benefits:
Efficient Data Handling: These tools enable organizations to process and analyze large datasets quickly and efficiently.
Advanced Analytics: They support complex analytics such as predictive modeling and real-time data analysis.
Challenges:
Complexity: Implementing and managing big data tools can be complex and requires specialized knowledge.
Cost: The infrastructure and resources needed for big data tools can be costly, particularly for on-premises solutions.
Data Integration Tools
Data integration tools are crucial for combining data from disparate sources into a unified view. They help in aggregating, cleansing, and transforming data, ensuring that it is consistent and usable for analysis.
Key Features:
Data Consolidation: Ability to gather data from various sources, including databases, cloud services, and APIs.
Data Transformation: Tools for cleaning, transforming, and enriching data to ensure consistency and accuracy.
Examples:
Talend: An open-source data integration tool that offers data extraction, transformation, and loading (ETL) capabilities, along with a user-friendly interface for data management.
Informatica: Provides a comprehensive suite of data integration tools for ETL, data quality, and master data management.
Apache Nifi: An open-source tool designed for automating data flow between systems, providing a flexible way to handle data ingestion, routing, and transformation.
How These Tools Facilitate Data Merging and Cleaning?
Data Merging: Data integration tools aggregate data from multiple sources, creating a cohesive dataset that provides a complete view of the information.
Data Cleaning: They automate the process of identifying and correcting errors or inconsistencies in data, ensuring that the analysis is based on high-quality information.
In summary, big data tools and data integration tools play a vital role in handling and preparing data for analysis, each addressing specific challenges associated with large-scale data management and ensuring that data is accurate and comprehensive for effective decision-making.
Future Trends in Data Analytics Tools
The integration of AI and machine learning (ML) into data analytics tools is transforming how data is analyzed. These technologies enable predictive analytics, automate data processing, and uncover insights that might be missed by traditional methods. Tools are increasingly incorporating AI capabilities for enhanced data pattern recognition and decision-making.
Automation in data analytics is on the rise, with tools offering advanced automation features for data cleaning, transformation, and reporting. This reduces manual intervention and accelerates the analytics process, allowing data scientists and analysts to focus on strategic tasks.
AI and ML algorithms can analyze complex data sets more efficiently and accurately, providing deeper insights and more precise predictions. This leads to more informed decision-making and better business outcomes.
AI-driven tools can offer personalized analytics based on individual user needs and preferences, tailoring reports and visualizations to specific requirements.
Cloud-based analytics tools are expected to become more prevalent due to their scalability, flexibility, and cost-effectiveness. Organizations are moving towards cloud solutions for real-time data analysis and storage.
As data analytics tools become more user-friendly, there will be a growing trend towards data democratization. This means making data access and analysis capabilities available to a broader range of users within organizations, not just data specialists.
Choose tools based on your specific data requirements, scalability needs, and budget.
Regularly update your knowledge on emerging trends and tools to leverage the latest advancements in data analytics.
Conclusion
As data analytics tools continue to evolve, driven by advancements in AI, automation, and cloud technology, staying ahead of trends is crucial for leveraging their full potential. These tools enhance analytical capabilities, streamline data processing, and democratize access to insights. For those looking to deepen their understanding and practical skills in data analytics, consider a Data Analytics Training Course In Noida, Delhi, Mumbai, Thane, Vadodara, Bhopal etc, offers valuable opportunities. This training will equip you with the expertise to navigate and utilize the latest tools and technologies, ensuring you remain competitive and effective in the ever-evolving field of data analytics.