Beyond the Certificate: Leveraging Additional Resources for Continued Learning in Data Science
- Vaishali pal
- Apr 25, 2024
- 3 min read
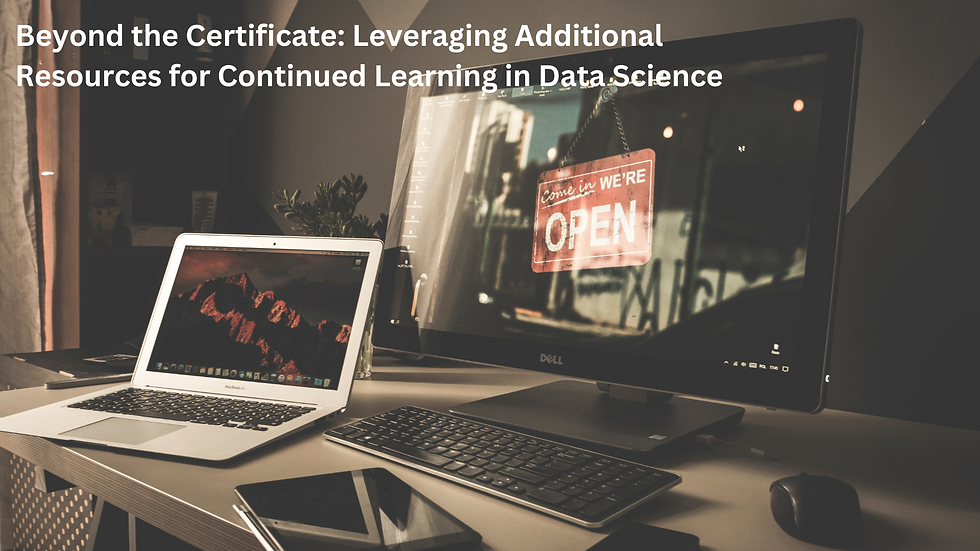
In the data-driven world of today, there is an increasing need for qualified data scientists. With the proliferation of data across industries, organizations are constantly seeking professionals who can derive valuable insights and drive strategic decision-making through data analysis. Consequently, many aspiring data scientists embark on a journey to acquire the necessary skills and credentials to excel in this field. While certificates and formal education are essential components of this journey, they are only the beginning. True mastery of data science requires a commitment to lifelong learning and the exploration of additional resources beyond traditional certification programs.
Certificates serve as a foundational stepping stone in the pursuit of a career in data science. They provide structured curriculum and validation of skills, which can be invaluable for entry-level positions or career transitions. However, the rapidly evolving nature of technology and the data landscape necessitate continuous upskilling and adaptation. Therefore, relying solely on certificates may limit one's growth potential in this dynamic field.
To truly excel in data science, individuals must embrace a multidimensional approach to learning that incorporates a diverse array of resources and experiences. Here are some strategies for leveraging additional resources beyond certificates to enhance your data science journey:
Online Courses and MOOCs: Supplement your certificate program with online courses and Massive Open Online Courses (MOOCs) offered by leading platforms such as Coursera, Udemy, and edX. These platforms offer a wide range of courses covering advanced topics in data science, machine learning, deep learning, and more. Additionally, they provide flexibility in scheduling, allowing you to learn at your own pace.
Open Source Tools and Libraries: Familiarize yourself with popular open-source tools and libraries commonly used in data science, such as Python, R, TensorFlow, and scikit-learn. Engage with the vibrant open-source community by contributing to projects, participating in forums, and exploring collaborative learning opportunities.
Practical Projects and Kaggle Competitions: Apply your knowledge and skills to real-world projects and Kaggle competitions to gain hands-on experience and exposure to diverse datasets and problem domains. Participating in Kaggle competitions not only hones your technical abilities but also fosters collaboration and innovation within a competitive environment.
Research Papers and Publications: Stay abreast of the latest research developments and breakthroughs in data science by reading research papers, academic journals, and industry publications. Engage with research communities through conferences, workshops, and online forums to expand your knowledge and network with experts in the field.
Networking and Mentorship: Build relationships with fellow data scientists, industry professionals, and mentors who can offer guidance, advice, and opportunities for growth. Attend networking events, meetups, and conferences to connect with like-minded individuals and learn from their experiences and insights.
Specialized Workshops and Bootcamps: Participate in specialized workshops, bootcamps, and training programs focused on specific areas of interest within data science, such as natural language processing, computer vision, or time series analysis. These intensive learning experiences provide concentrated knowledge and practical skills relevant to emerging trends and technologies.
Continuous Practice and Iteration: Dedicate time to continuous practice and iteration by working on personal projects, experimenting with new techniques, and refining your problem-solving abilities. Embrace a growth mindset that values learning from failures and setbacks as opportunities for improvement and innovation.
Industry Internships and Collaborations: Gain real-world industry experience through internships, freelance projects, or collaborative partnerships with companies and organizations. These opportunities provide invaluable insights into industry practices, challenges, and trends, while also allowing you to apply your skills in a professional context.
Cross-Disciplinary Learning: Explore interdisciplinary connections between data science and other fields such as business, economics, psychology, or biology. Drawing insights from diverse disciplines enhances your creativity, critical thinking, and ability to tackle complex problems from multiple perspectives.
Continual Reflection and Feedback: Reflect on your learning journey regularly and solicit feedback from peers, mentors, and instructors to identify areas for improvement and growth. Maintain a growth-oriented mindset that embraces lifelong learning as a journey of self-discovery and personal development.
In conclusion, while certificates, such as a Best Data Science certification course in Noida, Delhi, Mumbai, Bhopal & all other cities in India are valuable credentials in the field of data science, they represent just one aspect of a broader learning ecosystem. To truly thrive in this dynamic and evolving field, aspiring data scientists must embrace a holistic approach to learning that encompasses a diverse range of resources, experiences, and opportunities for growth. By leveraging additional resources beyond certificates, individuals can continuously expand their knowledge, skills, and expertise to excel in the ever-changing landscape of data science.